High-Quality Multi-View Partial Point Cloud for Completion
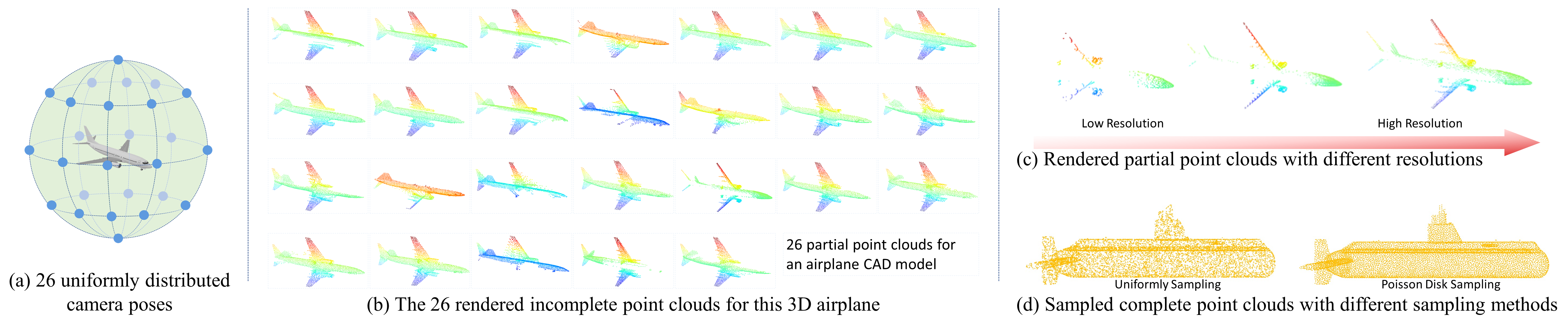
Multi-View Partial (MVP) Point Cloud Dataset. (a) shows an example for our 26 uniformly distributed camera poses on a unit sphere. (b) presents the 26 partial point clouds for the airplane from our uniformly distributed virtual cameras. (c) compares the rendered incomplete point clouds with different camera resolutions. (d) shows that Poisson disk sampling generates complete point clouds with a higher quality than uniform sampling.
News
2021-12-22 The workshop report is avaliable at Arxiv!
2021-10-17 Awards at ICCV2021 Workshop. Congradulations to all the winner teams!
2021-07-12 The submission on Codalab starts!
2021-07-10 The benchmark,
"Single-View Point Cloud Completion" has been released.
2021 The MVP challenges will be hosted in the ICCV2021 Workshop: Sensing, Understanding and Synthesizing Humans
.Details
Single-View Point Cloud Completion Benchmark evaluates the performance of generating complete 3D point clouds based on single-view partial point clouds. This is a large subset of MVP, containing massive high-quality incomplete and complete point clouds generated from 3D CAD models. It contains 104,000 number of partial point clouds and 4,000 number of complete point clouds from 16 number of object categories:
The Training set has 62,400 point cloud pairs, and their corresponding category labels.
The Testing set has 41,600 point cloud pairs, and their corresponding category labels.
The general pipeline is training your method on the Training set while evaluating on the Testing set. Note that the Training set and Testing set are the same with the data (2048 points) prepared in the paper VRCNet. Using the Testing set for training is strictly forbidden.
Download
You can also download data from Baidu Drive (code: p364).
Citation
@inproceedings{pan2021variational, title={Variational Relational Point Completion Network}, author={Pan, Liang and Chen, Xinyi and Cai, Zhongang and Zhang, Junzhe and Zhao, Haiyu and Yi, Shuai and Liu, Ziwei}, booktitle={Proceedings of the IEEE/CVF Conference on Computer Vision and Pattern Recognition}, pages={8524--8533}, year={2021} }
Contact
If you have any question, please contact Liang Pan at liang.pan@ntu.edu.sg.